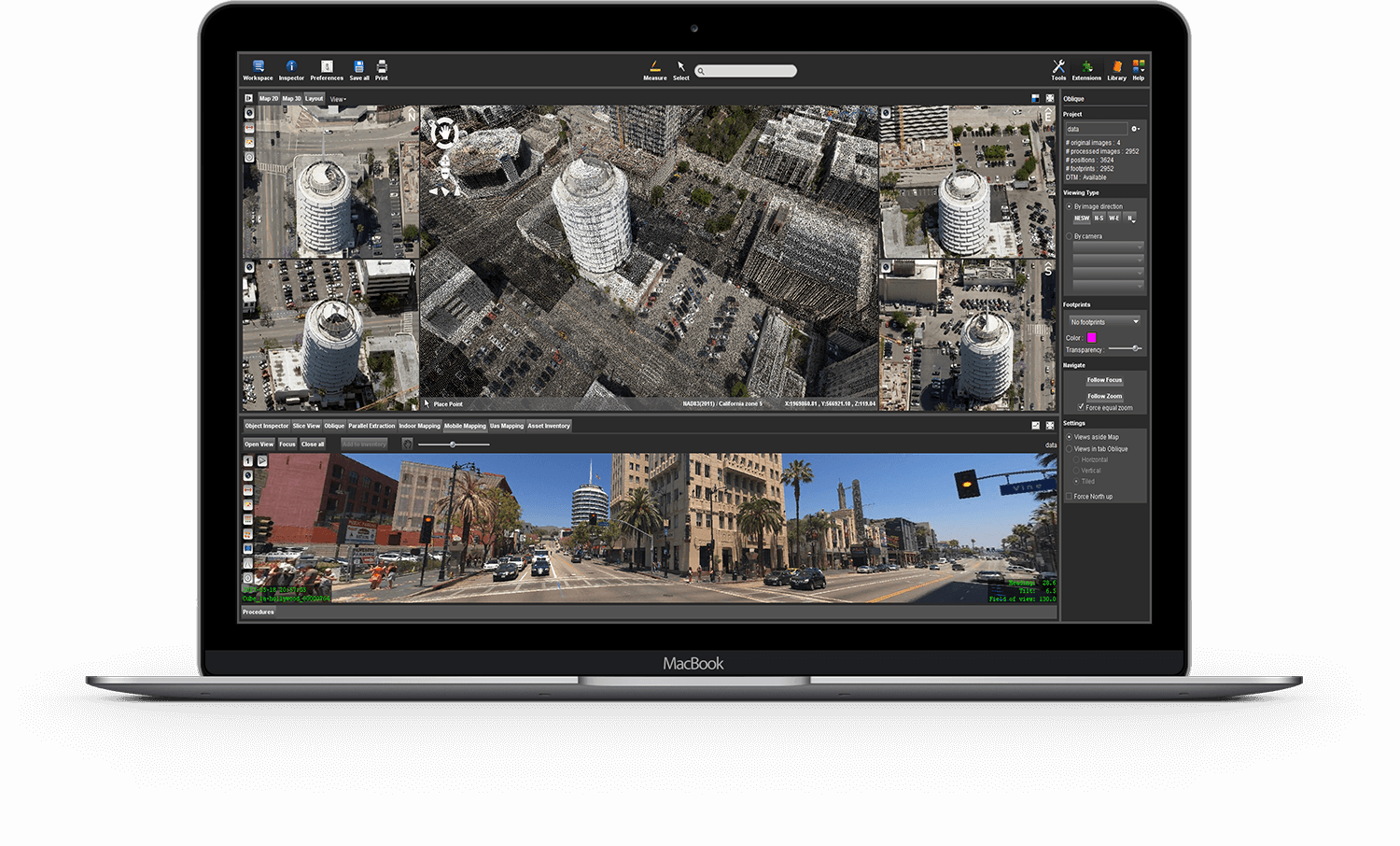
Haven’t we all been reading so many reports and views on this dilemma ? Didn’t we line up for panels and forums that discuss the topic ? Well, allow me to be disruptive : I don’t believe in a contradiction between both techniques, nor is one better than the other. They’re just techniques to achieve a certain goal. It’s the goal that matters, and the way to get there is subject to that need.
So both techniques deliver a point cloud. However, the attribution is different. Photogrammetry can provide an RGB value derived from its photogrammetry, but LiDAR can provide a reflectivity value, multiple returns, waveform information. The latter has more value for analysis of the registered points, while the first may result in a more dense point cloud, ready to be converted to a detailed mesh with sharp edges as these algorithms improve all the time. So what do you need : Pure mapping ? Photogrammetry is fine. Analysis ? Go for LiDAR.
In my humble opinion, the golden egg is the combination of both. Not so much in point cloud generation – obviously the errors involved in each capture technique might generate unwanted results that need post-processing and cleaning up – but the value of using an image while interpreting a point cloud is underestimated. In every job or project.
Whichever Reality Capture technique you use, it is imperative that you can interpret what you see. The human eye is used to see the real world and that is best represented by an image, not a point cloud. But the point cloud gives you depth, and if collected by LiDAR, a range of interesting information about each point. So the fusion of data gives you the best interpretation capabilities. Of course, this assumes a point cloud that covers all that we see in the image, and matches perfectly. When derived from imagery, matching is evident. When collected by separate sensors – a camera and a laser system – this is less obvious and requires attention. In streetlevel Mobile Mapping, this matching and alignment of sensors has long been solved and comes as an obvious advantage – though not all manufacturers deliver the same results. In other reality Capture techniques, this alignment may still be an issue. So again, it depends on what you want to achieve, what the needs of your job are, to be able to choose between photogrammetry and LiDAR. In any case, if you do LiDAR, do collect imagery in the same run or flight. With a fixed mount, lever arm corrections should be able to align image and LiDAR perfectly, even if the image is not used to derive a dense matched point cloud.
Photogrammetry has evolved so much in the last decade that one can hardly recognise it. People tend to associate it with the dense matching process, whilst forgetting that the fundamental techniques are being used in many more applications, even in Apple’s ARkit and Google’s Tango project. In the Mapping business, it is predominantly present in the UAS world, as payload is key to the flight time of drones, and there are good low-weight cameras to be found compared to LiDAR systems that require more lifting power. Also there we’ve seen an evolution from very low end camera’s being mounted on fixed wing drones resulting in nothing better than images subject to motion blur, to quality cameras and GPS fixed rotary wing drones that deliver very sharp images. Dense matching techniques have evolved from generating a bell-shaped house to structures with sharp edges, and more-over the result is not only a point cloud but even so a textured mesh of the same area. That on its turn is the advantage of having a dense point cloud, something that is much harder to achieve with LiDAR systems.
Engineers no doubt prefer hands-on with the point cloud, however, it is my strong belief that the same data, if collected properly and perfectly aligned, can provide superb input in numerous workflows for the non-specialist. Use the image as the user interface, and provide the point cloud as invisible depth generator – on the fly of course. However you generate your data, it will have a second life and provide answers to questions of many people, far beyond the initial job that invoked the data collection.
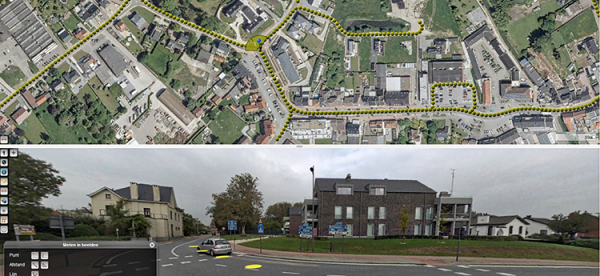
Flemish Government maps all streets
Read More